EnvirometriX is now providing professional service to support soil sampling for Soil Organic Carbon (SOC) monitoring. We have developed a unique service for optimal sampling and re-analysis of the SOC stocks for a given year of interest. To request support with organizing monitoring of the soil carbon, please contact us directly.
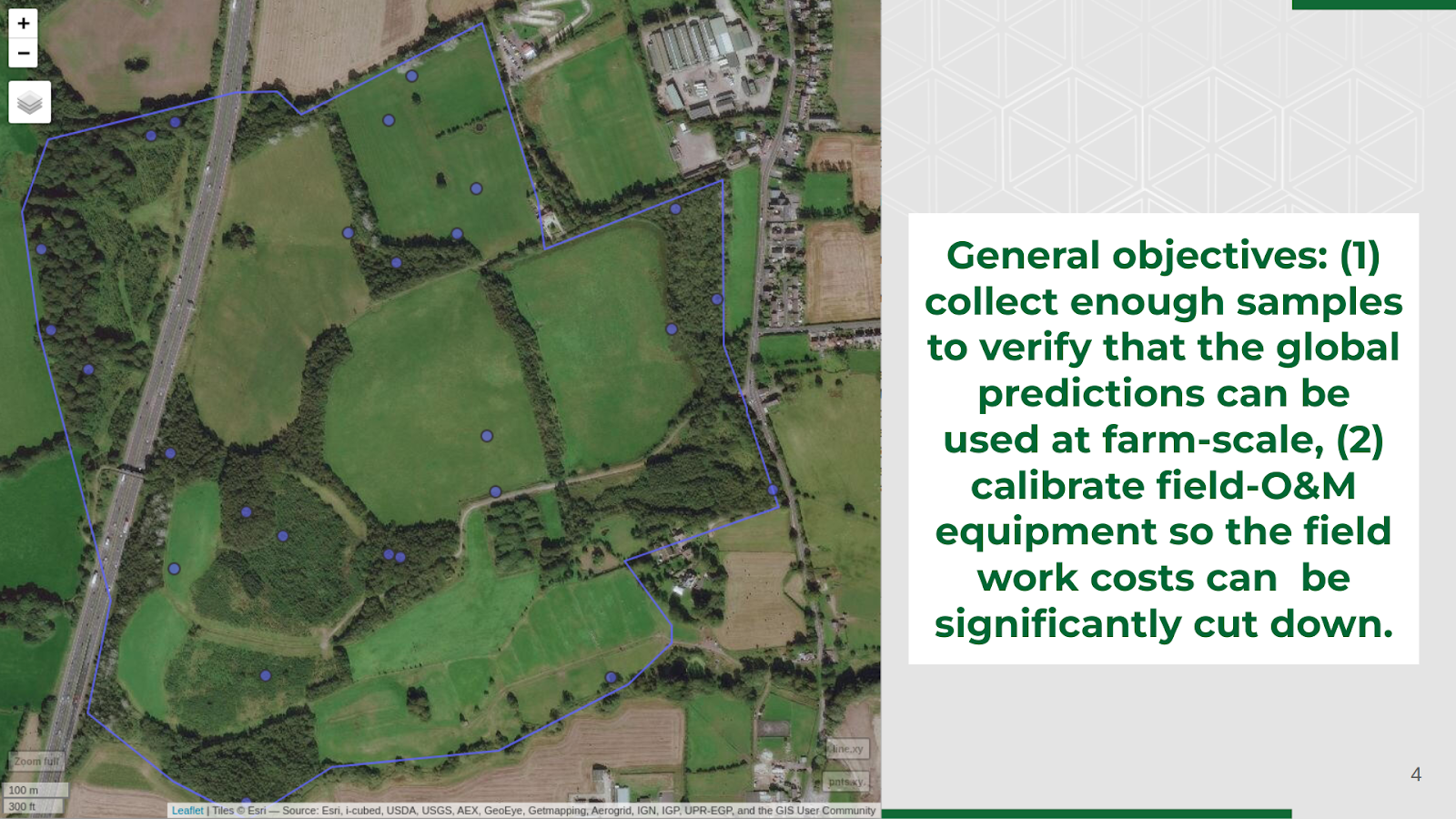
How does the sampling protocol works?
The proposed methodology for sampling is based on uncertainty-guided sampling strategy (Stumpf et al, 2017) where SOC samples are distributed proportionally to the probability of initial prediction errors exceeding the threshold error. This approach is selected because it focuses on decreasing uncertainty of predictions of SOC and hence maximizing tradable SOC. It also avoids the user having to make possibly arbitrary decisions on covariates for geographical stratification.
In the case of uncertainty-guided sampling points are allocated based on the initial errors estimated using globally predicted values for SOC (based on a spatiotemporal model) and are specific to the year of sampling. The uncertainty-guided sampling can be best described with the following four generic steps:
- Survey #1: produce initial sampling design using enough points required to calibrate the global model (recommended sampling algorithm: Feature Space Coverage Sampling).
- Collect measurements of SOC at sampling locations, re-calibrate the global spatiotemporal model (spacetime-SOC) and re-estimate predictions and prediction errors for the farms.
- Fit a model explaining drop in prediction errors given sampling intensity and environmental conditions. Use the model to estimate the optimized sampling plan for survey #2.
- Collect measurements of SOC at sampling locations, re-calibrate the global spatiotemporal model (spacetime-SOC) and re-estimate predictions and prediction errors for the farms. Estimate SOC fluxes and connected uncertainty.
- Repeat from step 2 to 4 until sufficiently accurate predictions of SOC can be produced using only EO data.
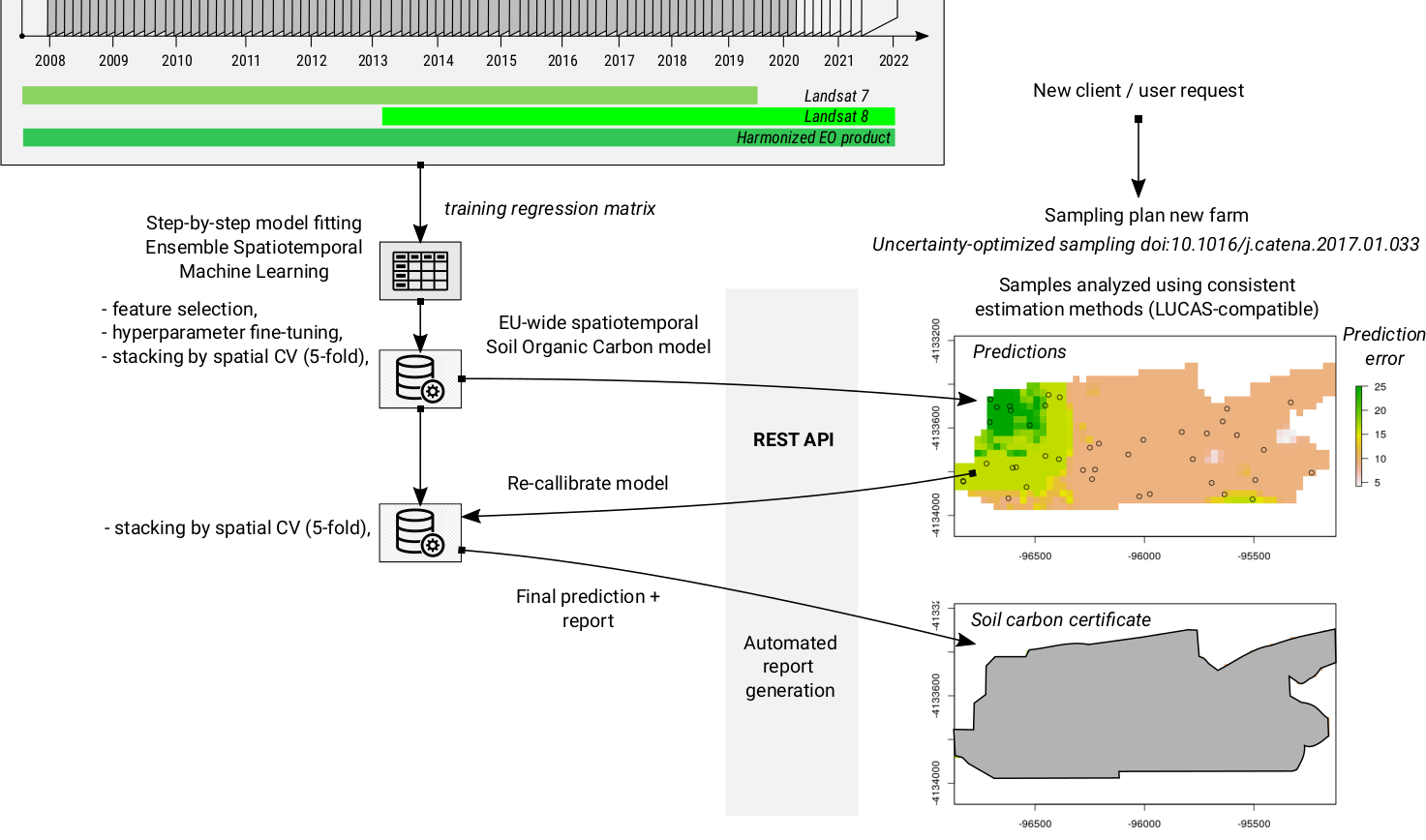
How does spatiotemporal Machine Learning works?
In the case of the spatiotemporal Machine Learning (ML), predictions are generated from models where ground observations (points) are overlaid by matching the season/year of sampling with time-periods of covariates. Covariates include monthly, annual, accumulated and derivative products, hence accurately represent accumulation and deposition processes.
The main advantages of uncertainty-based sampling for spatiotemporal Machine Learning are:
- Cost-reduction: Sampling costs can be significantly reduced. In fact, our estimate is that already 3–4 new samples could help significantly drop prediction errors e.g. 20–30% for the specific plot. This assumes, however, quality sampling and no noise in the point data. The lower the prediction errors the more SOC [t/ha] can be traded of course.
- Bias reduction: Local sampling at selected farms, then reanalysis of the global models eventually helps reduce bias and increase accuracy also for pan-EU i.e. the whole continent.
- Consistency: When estimates of SOC are based on pan-European models then this ensures that the predictions and estimates of SOC fluxes are consistent across the whole continent. This allows users to compare performance of agricultural farming practices across different time-zones i.e. without a need for harmonization / re-calibration.
- Causality testing: Because background data (data cube) is extensive and because the models are spatiotemporal, they can also be used to try to explain causality i.e. causes or main drivers of changes in SOC. For example, our EnvirometriX SOC API shows how SOC drops are highly correlated with drops in NDVI and vice versa. This is just one example that could be further sophisticated and extended to hundreds of variables.
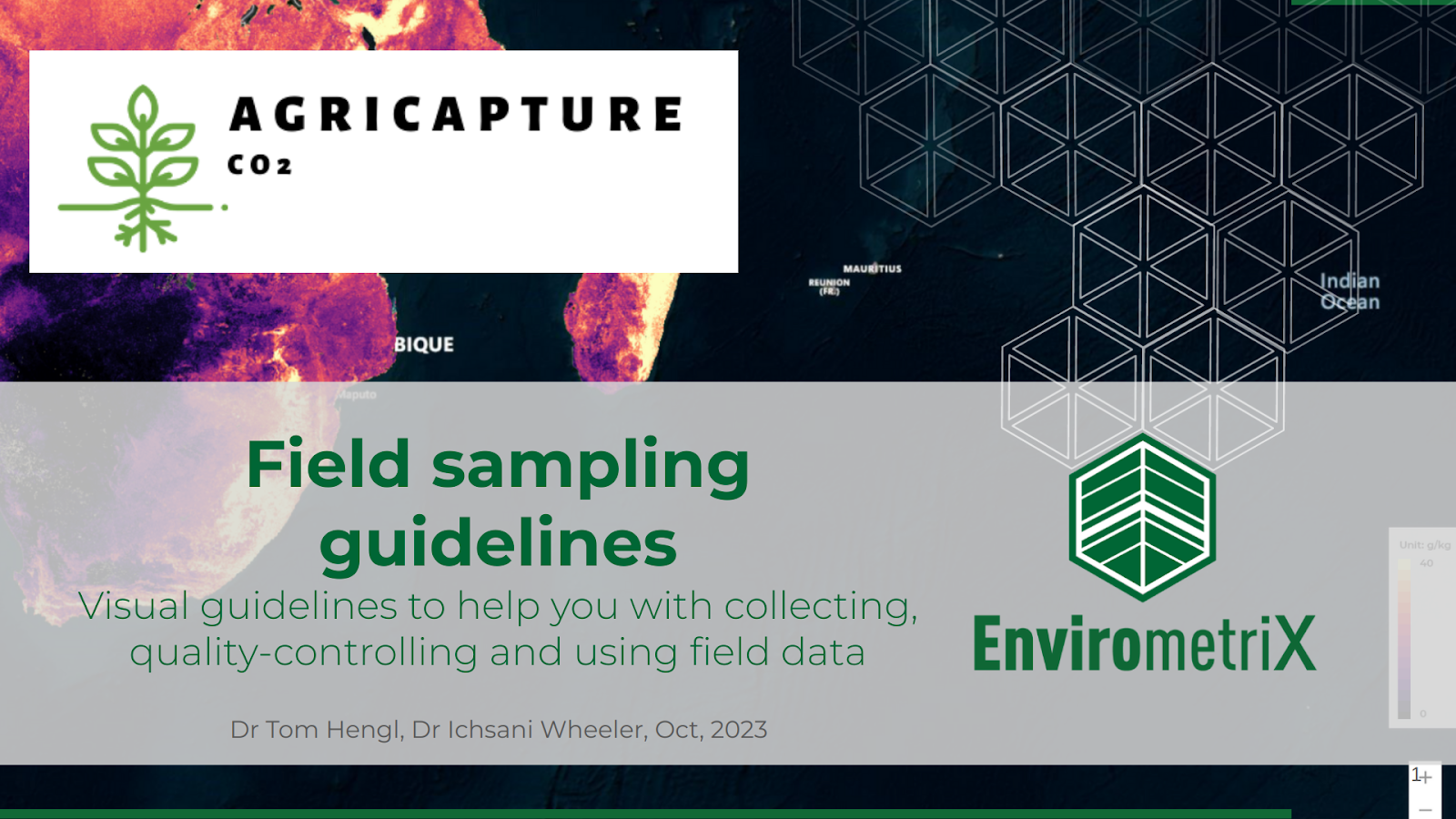
Standard SOC services that EnvirometriX provides (based on the AgriCapture project) include:
- Generation of soil sampling designs based on various optimization criteria (usually aiming at minimization of the prediction errors / uncertainty in estimates and/or costs),
- Standardization, harmonization and calibration of values: from soil organic carbon content estimates [%] based on various methods, to estimates of soil organic carbon stocks [t/ha] and below-ground biomass,
- Estimation of baseline / time-specific soil organic carbon stocks across scales (field to region to continent),
- Derivation of the SOC change and confidence intervals connected with derivation of 𝜟SOC,
- Estimation of SOC for large areas at high spatial resolution (e.g. 10-m to 30-m) with per-pixel uncertainty,
- Estimation of SOC sequestration potential, including SOC under various short- and long-term scenarios (e.g. 5, 10, 20, 50-yrs in advance) including the climate change scenarios,
Read more about soil carbon sampling and estimation in:
- de Gruijter, J. J., McBratney, A. B., Minasny, B., Wheeler, I., Malone, B. P., & Stockmann, U. (2018). Farm-scale soil carbon auditing. In Pedometrics (pp. 693-720). Springer, Cham. https://doi.org/10.1007/978-3-319-63439-5_23
- Hengl, T., Sorenson, P., Parente, L., Cornish, K., Battigelli, J., Bonannella, C., … & Nichols, K. (2023). Assessment of soil organic carbon stocks in Alberta using 2-scale sampling and 3D predictive soil mapping. FACETS, 8, 1-17. https://doi.org/10.1139/facets-2023-0040