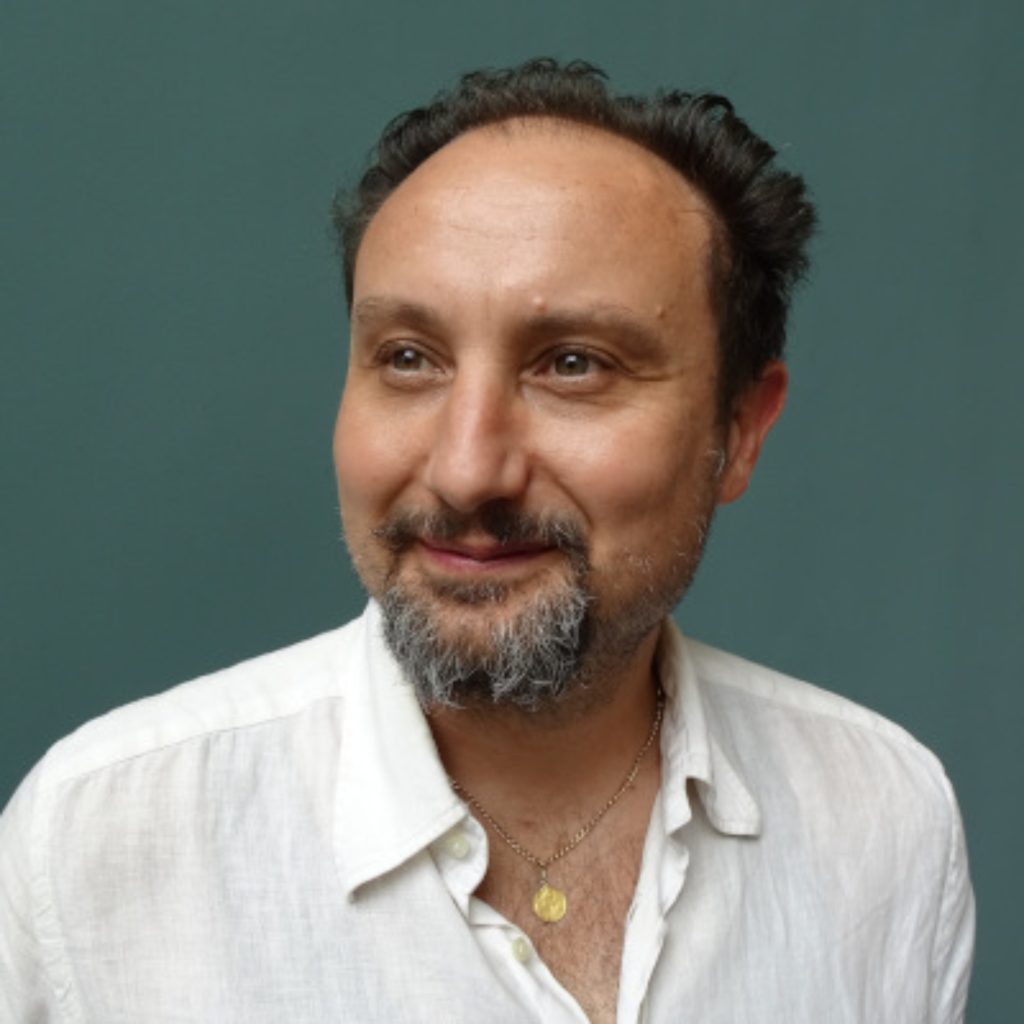
Tomislav Hengl
Co-Founder
Tom Hengl has more than 20 years of experience as an environmental modeler, predictive soil mapper, data scientist and spatial analyst. He has collaborated on projects with numerous international organizations including The Nature Conservancy, Woods Hole Research Centre, the Bill and Melinda Gates Foundation, UNCCD, Africa Soil Information Service, PBL and numerous other organizations.
His extensive experience in spatial prediction and statistically-based modeling has led him to become a widely recognized expert in the areas of Machine Learning and Big Geodata for environmental modeling.
Phone: +31 (0) 317 427537
Email: tom.hengl@envirometrix.net
Education:
- Post-doc, IBED / University of Amsterdam (Ecology / biodiversity)
- Post-doc, JRC European Commission (Soil science / Pedometrics)
- PhD, ITC / Wageningen University (Geoinformation science)
- MSc, ITC Enschede (Soil Resource Management)
Appointments:
- 2018–present: Vice-Chair OpenGeoHub Foundation
- 2018–present: EnvirometriX Ltd., The Netherlands.
- 2010–2018: Senior researcher, Wageningen University / ISRIC, The Netherlands.
- 2007–2010: Post-doctoral researcher, University of Amsterdam, The Netherlands.
- 2005–2007: Post-doctoral researcher, European Commission JRC, Italy.
- 1998–2005: PhD student / Ass. Professor, Croatia / The Netherlands.
- Machine Learning
- Global soil and vegetation mapping
- High Performance computing
- Hengl, T., MacMillan, R.A., (2019). Predictive Soil Mapping with R. OpenGeoHub foundation, Wageningen, the Netherlands, 370 pages, www.soilmapper.org, ISBN: 978-0-359-30635-0.
- Hengl, T., Nussbaum, M., Wright, M. N., Heuvelink, G. B., and Gräler, B. (2018) Random Forest as a generic framework for predictive modeling of spatial and spatio-temporal variables. PeerJ 6:e5518.
- Sanderman, J., Hengl, T., Fiske, G., (2017) The soil carbon debt of 12,000 years of human land use. PNAS, doi:10.1073/pnas.1706103114
- Ramcharan, A., Hengl, T., Nauman, T., Brungard, C., Waltman, S., Wills, S., & Thompson, J. (2018). Soil Property and Class Maps of the Conterminous United States at 100-Meter Spatial Resolution. Soil Science Society of America Journal, 82(1), 186–201.
- Hengl, T., Leenaars, J. G., Shepherd, K. D., Walsh, M. G., Heuvelink, G. B., Mamo, T., et al. (2017) Soil nutrient maps of Sub-Saharan Africa: assessment of soil nutrient content at 250 m spatial resolution using machine learning. Nutrient Cycling in Agroecosystems, 109(1), 77–102.
Hengl T, Mendes de Jesus J, Heuvelink GBM, Ruiperez Gonzalez M, Kilibarda M, Blagotic A, et al. (2017) SoilGrids250m: Global gridded soil information based on machine learning. PLoS ONE 12(2): e0169748. doi:10.1371/journal.pone.0169748